报告一时间:11月5日,上午10:00-11:30
报告二时间:11月6日,下午15:30-17:00
报告地点:航空楼A706
邀请人:黄玮副教授马玉娥教授
报告人简介:
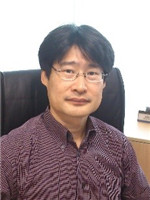
Prof. Yong-Wei ZHANG is Principal Scientist II and Deputy Executive Director at Institute of High Performance Computing (IHPC), A*STAR, Singapore. He is Adjunct Professor at both National University of Singapore and Singapore University of Technology and Design. His research interests focus on using theory, modelling and computation as tools to study the relationship between structures and properties of materials, materials design, materials growth and manufacturing, property engineering, mechanical-thermal coupling, mechanical-electronic coupling, and mechanical properties of biomaterialsetc. He has published over 450 refereed journal papers, and delivered over 80 invited/keynote/plenary talks and lectures. He received Best Paper Award (2011-2016) and Highly Cited Paper Award (2014, 2015, 2017) from Institute of High Performance Computing, A*STAR; and A*STAR Aerospace Programme Achievement Award (2013). He is listed as Global Highly Cited Researchers (2018) by Clarivate Analytic. He is Editorial Board Member forAdvanced Theory and SimulationandInternational Journal of Applied Mechanics.
报告一
报告题目:
An integral digital twin platform for powder-bed fusion additive manufacturing: From powder to part
报告内容:
Part property inconsistency and quality control are two major bottleneck issues in the current additive manufacturing (AM) technology [1]. Computer simulation is a powerful tool to gain in-depth understanding into these bottleneck issues [2]. We have developed an integrated digital twin platform for powder-bed selected laser melting (SLM) with aim to predict the printing outcomes, such as porosity, grain and phase microstructures, residual stress distribution and distortion, surface roughness and mechanical properties for given printing conditions. In this platform, a discrete element method is used to describe powder bed packing and levelling, a ray-tracking method is used to predict the laser energy adsorption, a combined lattice Boltzmann-phase field method is used to describe the melt pool dynamics, solidification process and solid phase transformation, and a homogenization method is used to estimate the mechanical properties. We have used IN718 super-alloy as a model material to test the predictability of the platform. Our simulation results show that the predictions are able to reproduce many interesting features of SLM processes. It is expected that the developed digital twin platform is of the potential to significantly promote the adoption of additive manufacturing technology by industry.
报告二
报告题目:
High throughput computation and machine learning-aided design for high entropy alloys
报告内容:
There are constant needs for new materials of higher performance and lower cost since new materials discovery and development is able to strongly drive economic growth.New materials developments are, however, costly, lengthy and empirical. The materials development cycle from discoveryàdevelopmentàoptimizationàintegrationàcertificationàmanufacturingàdeployment often takes ~ 20 years. High Entropy Alloys (HEAs) were discovered recently. However, the composition space for HEAs is vast. Recent studies have shown that HEAs can exhibit rich microstructures, for example, in solid solution, single/multiple phases, precipitates, nanocrystals and amorphous phases, etc. They can also show superior and novel mechanical properties, which may be promising for many important applications, such as (i) light-weight materials for aerospace and transport, (ii) high-temperature and heat-resistant components in turbines and (iii) super hard materials for machining and tooling. Given the wide materials and application space to explore, an interesting question is: Can we employ high-throughput computation and machine learning to accelerate new HEAs developments from discovery to deployment? Recent breakthroughs in high-throughput computing techniques and machine learning techniques present a brand-new opportunity to tackle this issue. To make this happen, we need to have a large material database for HEAs. However, the existing database for HEAs is still very limited. Hence, we propose to develop a machine-learning-guided approach to accelerate the discovery, development and optimization of HEAs by leveraging onDFTcalculations, CALPHADcalculations; and machine learning. In this talk, we will present some of our recent developments on these research aspects.